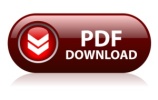
Necula Sabina-Cristiana
PhD in Economics, Scientific Researcher,
Department of Research,
Faculty of Economics and Business Administration,
Alexandru Ioan Cuza University of Iasi, Romania,
11 Carol I Blvd., Iasi, 700506, Romania
sabina.necula@uaic.ro
Perspectives on the use of deep learning
in business
Abstract: This paper presents the perspectives on the use of deep learning in business domain. It treats the actual context, the traditional decision support tools and fucntionalities and the present deep learning models possible to use in business. It actually raises the main points of interest of today’s business world always dynamic, always online, dealing with lots of technologies and lots of data. Artificial neural networks are designed such that they can identify the underlying patterns in data and learn from them. They can be used for various tasks such as classification, regression, segmentation, and so on. This paper treats the problems that decision-makers addresses with deep learning models.
Keywords: deep learning, decision support systems, machine learning, business, data mining.
JEL Classification: M15, O33, O32.
Bibliography
ACKOFF, R. L., 1967. Management Misinformation Systems, Management Science, 14(4), pp. 147-156, http://ackoffcenter.blogs.com/ackoff_center_weblog/classics/index.html
AIRINEI, D., 2006. Sisteme de asistare a deciziilor, Iasi: Sedcom Libris.
ANDONE, I., TABĂRĂ, N. (coord.), 2006. Contabilitate, tehnologie şi competitivitate, Editura Academiei Române, Bucureşti, p.323
CERNY, P. A., 2017. Data mining and Neural Networks from a Commercial Perspective, http://citeseerx.ist.psu.edu/viewdoc/download?doi=10.1.1.117.1820&rep=rep1&type=pdf
CODD, E. F., CODD, S. B., SALLEY, C. T., 1993. Providing OLAP to User-Analysts: An IT Mandate, E.F. Codd Associates.
FOTACHE, D., HURBEAN, L., 2004. Integrated Systems for Business management- Enterprise Resource Planning (Sisteme integrate pentru gestiunea afacerilor – ERP), Bucureşti: Editura Economică.
HAAG, S., CUMMINGS, H., PHILLIPS, M., 2006. Management Information Systems for the Information Age, New York: McGraw-Hill Irwin, Inc.
HSU, A., 2017. Deep Learning vs. Machine Learning for Business Outcomes: A Data Scientist’s Perspective, https://insidebigdata.com/2017/10/27/deep-learning-vs-machine-learning-business-outcomes-data-scientists-perspective/
HUBER, G. P., 1984. Issues in the Design of Group Support Systems, MIS Quarterly.
HURBANS, R., 2017. Artificial Intelligence in Perspective, https://medium.freecodecamp.org/artificial-intelligence-in-perspective-6331dc384685
HURBEAN, L., 2005. Performance Management with Business Intelligence, The Impact of European Integration on the National Economy Conference, Cluj-Napoca, Editura Risoprint.
IMTIAZ, S., BRIMICOMBE, A. J., 2017. A Better Comparison Summary of Credit Scoring Classification, International Journal of Advanced Computer Science and Applications, Volume: 8, Issue: 7, pp. 1-4.
KIMBALL, R., 1998. Brave New Requirements for Data Warehousing, Vol. 1, Nr.1, p. 12.
KINGSLEY, M., 2016. Artificial Intelligence: How will it affect legal practice – and when? https://blogs.thomsonreuters.com/answerson/artificial-intelligence-legal-practice/
MALIK, M. S. I, HUSSAIN, A., 2017. Helpfulness of product reviews as a function of discrete positive and negative emotions, Computers in human behaviour, Volume: 73, pp. 290-302.
MORA, M., FORGIONNE, G., GUPTA, J., 2002. Decision Making Support Systems: Achievements and Challenges for the New Decade, Idea Group Inc.
NICOLESCU, O., 2001. Sistemul informaţional-managerial al organizaţiei, Bucureşti: Editura Economică
O’BRIEN, J., MARAKAS, G. M., 2006. Management Information Systems, 7th Edition, McGraw-Hill International.
POWER, D. J., 2007. A Brief History of Decision Support Systems, editat de DSSResources.COM, http://dssresources.com/history/dsshistory.html
XIAO, J., CAO, H., HANWEN, X., JIANG, ET AL., 2017. GMDH-based semi-supervised feature selection for customer classification, Knowledge Based Systems, Volume: 132, pp. 236-248.
ZHU, B., NIU, Y., XIAO, J., ET AL., 2017. A new transferred feature selection algorithm for customer identification, Neural Computing & Applications, Volume: 28, Issue: 9, pp. 2593-2603.